Distributional models for lexical semantics
Course author
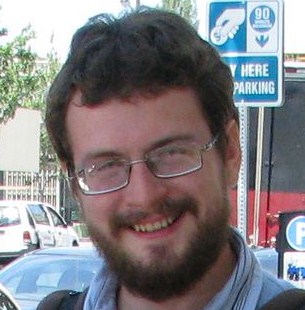
Researcher, Lorraine Laboratory of Computer Science and Its Applications (Loria), CNRS, University of Lorraine
Course materials
Presentations
slides_1.pdf slides_2.pdf slides_3.pdf
Assignments
assignment1.pdf assignment_1_solution.pdf
assignment2.pdf assignment_2_solution.pdf
References
Course annotation
The course will discuss vector-based distributional semantic models and their application to linguistic problems.
Distributional semantic models, also known as word embeddings, are an increasingly popular tool in computational semantics. In a distributional model, each word (or other linguistic expression of interest) is represented as a multidimensional numeric vector. Vector operations such as addition, pointwise multiplication, or linear maps can serve as analogs of semantic composition operations for vector based representations.
Comparisons between vectors are used to predict various semantic relations between words, such as semantic relatedness or hyperonymy, various psycholinguistic measures such as the degree of semantic priming between words, and, lately, diachronic lexical semantic development and lexical semantic typology.